Pod ran out of CPU RAM
I somehow managed to run out of RAM (not VRAM, system RAM)... right after a very compute-heavy operation (calculating quantized KV-Cache scales)... while running
model.save_pretrained
... while the weights are still in VRAM... The pod is still running, but completely unresponsive.
Now that you're done laughing at my misfortune, is there anything at all I can do to save those weights? Even enabling some swap would be completely fine... I just want the weights to save to the networked drive...
Pod ID: tybrzp4aphrz3d351 Replies
You should contact support on their website without terminating the pod
OK - thanks. Hopefully they get back to me soon...
If the process got killed, there is no way to recover data soo
I know that the process is alive and the data is still stored in VRAM. I ran into similar issues with local containers that ran out of memory, simply adding some memory (whether it's RAM or swap) will immediately bring it back to life. It's merely thrashing as it tries to clear the disk cache while new data is being written to.
Still don't know how it managed to eat that much, the weights are 140GB and I have 283GB of RAM...
Wow if its a H100 you are burning money fast
Hope support reaches you soon
It's a B200. I'm burning more money than I'd like.....
Lol
It would be very funny if it wasn't my pod lol
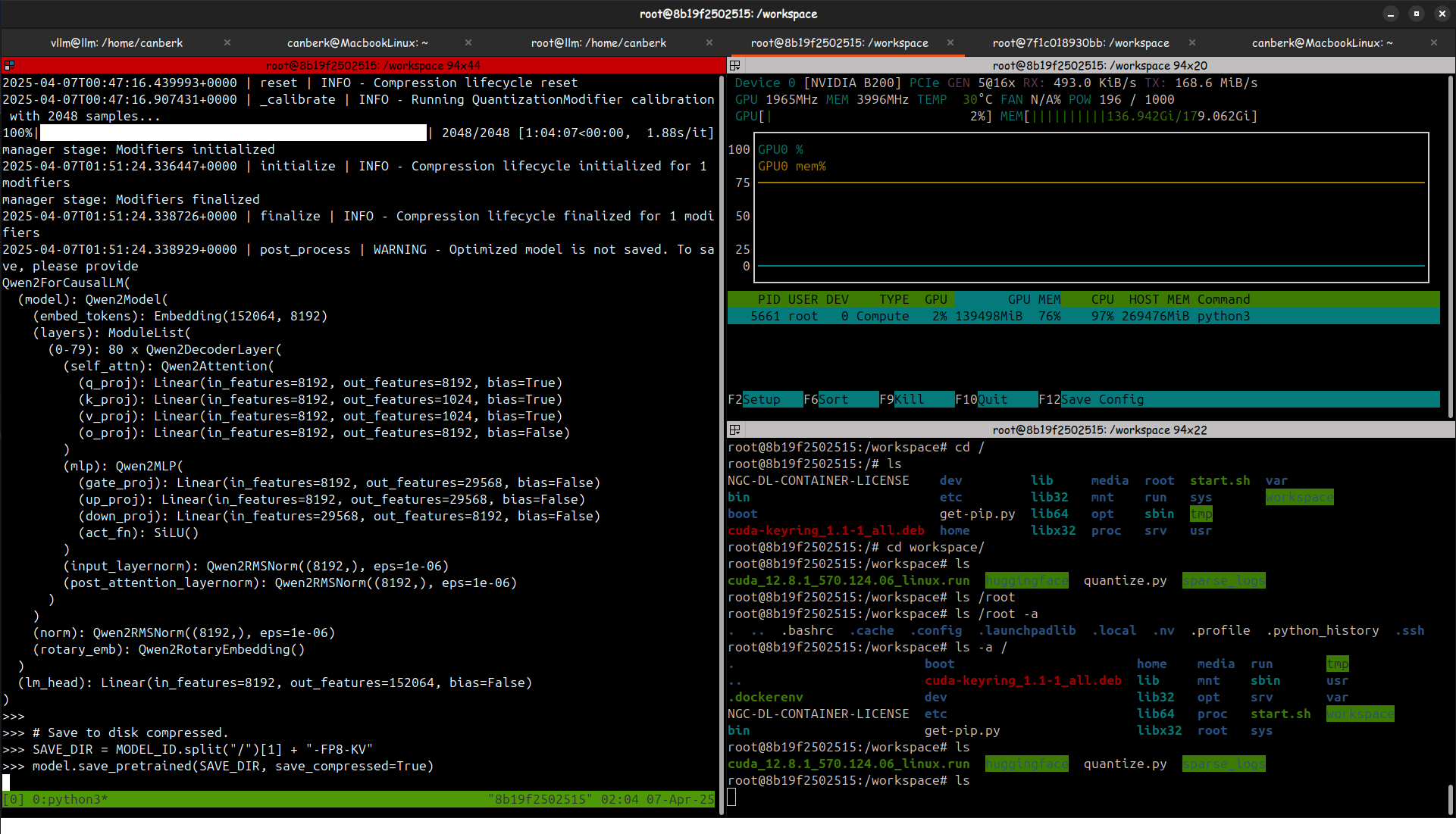
Maybe 2 instance of model loaded to system ram?
That's very possible. I guess it might be trying to load it to RAM while it writes to disk or something
Sad part is that the file I want is only a few megabytes, but the only way to get it is to call
model.save_pretrained
Ohh you running quantization?
Not quite. I'm calculating quantized KV scale factors. The idea is to be able to quantize the KV-cache down to 8 bits while losing very very little in accuracy.
You can take out an extra bit from the exponent, making kv-cache weights e4m3 (with one sign bit) instead of e5m2. However, this destroys the numerical range in which you can represent weights, since you just removed a whole bit from the floating point exponent. If you happened to have some magic numbers you can multiply each weight by, calibrated by running thousands of inference without any quantization on a very powerful GPU... You still wouldn't quite get to non-quantized quality, but you'd get quite close
yeah saw that on vllm docs
Unfortunately I do not have 140GB of VRAM at home to go calculate my own scale factors lol
doesnt it work on cpu?
... I don't have 140GB of RAM, either. It's also painfully slow on CPU, and Flash Attention won't work. AFAICT Qwen really wants Flash Attention - people are saying the model breaks pretty badly without it
maybe the "running thousands of inference without any quantization on a very powerful GPU" part is a bottleneck
if you can run it on CPU you can always rent some high mem machines
It's not very different than simply running the model a few thousand times. But that's not very fast when you are running a 70b at full precision lol
yeah
Apparently they're based in New Jersey, and it's 11:30PM there
... maybe I should just stop the money burning
i think so too
Well, I tried lol
I have a question: if it is running inference over and over can it do the calibration layer by layer?
It can, actually, yeah
That's a very good point
Qwen 2.5 has 80 layers... One layer at a time would probably easily fit on a GPU I have at home
probably hurts to implement tho (if there is no implementation)
There is definitely no implementation. Even the code in vllm's docs are broken lol
I had to modify it to get it to work at all
Then I had the rude awakening of "you can't do this with a quantized model"... and here we are
lol
no multi-gpu implementation too?
Nope
sad
Well, maybe actually
Not that it matters with 140GB of weights lol
GitHub
GitHub - vllm-project/llm-compressor: Transformers-compatible libra...
Transformers-compatible library for applying various compression algorithms to LLMs for optimized deployment with vLLM - vllm-project/llm-compressor
They just pass in an
AutoModelForCausalLM.from_pretrained
model to the libraryhttps://github.com/vllm-project/llm-compressor/issues/965
maybe this is simillar to your case
GitHub
The new version 0.3.0 takes a long time for quantization and eventu...
Describe the bug I used the sample code (W8A16) to quantize THUDM/glm-4-9b-chat-hf on an Nvidia 4090, and the entire process was very slow (nearly 24 hours), with extremely high memory usage, to th...
Interesting - that allows multi-GPU. I wonder if I could implement some sort of per-layer processing...
It would be miserably slow for sure, especially since I can't do much batching without seriously modifying the library
GitHub
llm-compressor/examples/big_models_with_accelerate/README.md at mai...
Transformers-compatible library for applying various compression algorithms to LLMs for optimized deployment with vLLM - vllm-project/llm-compressor
Hmm... combining them all, I just might be able to fit it in a lot of small GPUs... saving lots of money. Probably still un-doable at home though since I don't think I can pass a quantized model through at all
Thank you!
try 4x A40s
192GB VRAM and about 1/6 of B200 pricing
How can one get more RAM in runpod?
even if it's swap
My understanding is that because I'm in a container, I can't just add in swap
Same for me
GitHub
OOM during save_pretrained of compressed model Ā· Issue #1183 Ā· vl...
Describe the bug The OOM was for CPU RAM. GPU RAM usage was normal, the model takes up less than half of the GPU. This was hitting the llmcompressor's modified save_pretrained_wrapper from llm-...
same issue
looks like quanting on cpu should be possible
Yes, I can see the same frustration in the comments section lol
Maybe I'll just go make an EC2 instance with a lot of EBS storage, enable swap, and go away for a month lol
Probably cheaper...
try these
Hm? I didn't see any suggestions in the GitHub issue
they are cheap with spot requests
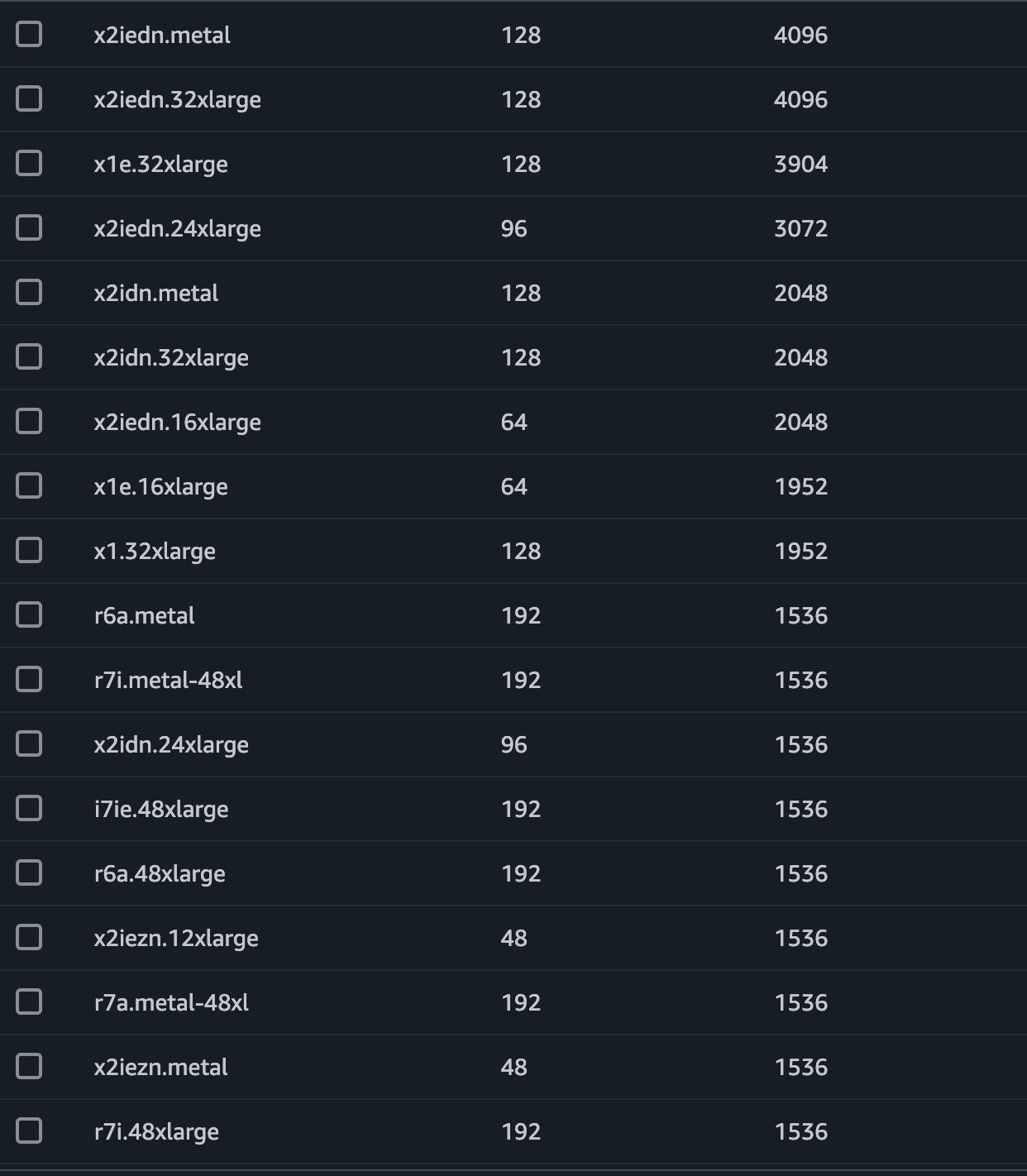
no GPUs tho
I suspect if I'm going CPU, I can go much much cheaper
yeah and if you are going spot, look for spot savings=90%
noone is using them and they dont get terminated as often
Spot seems iffy. I use AWS at work and have been evicted before - especially for long-running workloads
But nothing stops me from getting like a c7a.medium for a month, just letting it churn all day all night, with some EBS as swap
thats right
and go with instance store rather than EBS if that's possible
That's true - it's gonna be a lot faster
NVME powerr
š
Yes lol
Anyway thanks a lot for your help! Although the results are gone, hope my mistake at least gave people a laugh lol
I have the same experience with 70B models on a H100
can relate
Filter out when you create a pod
Well I feel kind of sad for your lost of progress
I... couldn't find enough RAM. Maybe it speaks to my horrifying setup, but the pod I was on had 260 something gigabytes and I OOM'd it...
I do too, but such is life. I want to re-run it regardless but I need a genuinely stupid amount of RAM to assure myself this will never ever happen again lol
Hmm I think the only way is More gpus
My guess is that torch tried to copy the weights to RAM... twice. No idea why it would happen. Seeing I am working with a 72b at bf16, that's 288+GB of RAM I need
Or other gpu types
I had a B200, VRAM isn't an issue. System RAM is
It can even be swap tbh, but since I'm in a container, I can't have my own swap. Someone has to give it to me during the docker run command. And runpod doesn't have such an option sadly...
Yeah I mean try sliding right that gpu count slider
And then you'll see the pod will have more ram, we'll if you use it for ram only it'll be waste too
That's a good point
I could get lots of cheaper GPUs and tensor parallelize, but the RAM was what killed my workflow from the start
I think there is shm
In /dev/shm, don't know if that's usable for you
Check your training script again heheh
I suspect not. It's all abstracted away from me - torch is what eats the RAM
the line that killed my pod was
model.save_pretrained()
and it's hard to avoid that lolCan chatgpt provide with a reasonable explanation of why
Maybe it can explain hf's code lol
Possibly lol I should ask after work
Maybe got to do with training and then saving it
The training code is rather brief - nothing crazy, some open source code from vllm that I modified to work with another LLM
It's not even training code - it's quantization code
How long did it took you?
Ic
Calibrating?
A few hours on a B200, with another few spent on various failures
I'm getting scales for a kv-cache quantization. I run LLMs at home and I need to quantize my kv-cache down to 8bpw with minimal loss
https://docs.vllm.ai/en/latest/features/quantization/quantized_kvcache.html except the example code is broken
Ways to go bankrupt fast š¦
Oh people actually do use high-end gpu for that
You need to run inference on unquantized model for it... and I am running a 72b at home
Aws does it on a 8xH100
You don't need a high-end GPU for vllm. Well, I don't. But I have an insane setup lol
Ooh
For which of their models
This runs qwen 2.5 72b with 14k context on 2x3090, with the nice PagedAttention that lets you serve many people at once:
What about the new llama oh wait even a pair of 4090 doesnt run it
That's cool
Too big and not worth the extra VRAM. 104B for what roughly benchmarks the same as qwen 2.5/llama 3.3
Ohh
I could squeeze even more quality out of this poor server if I could get the KV-cache scales
Unfortunately for that I need to put a lot more money in my account lol
Maybe next paycheck... If I can sanely get the RAM...
I have a question
Why do you need to run a fill model for that kv cache scaling
No other people had even did this for qwen before?
If you are running a quantized model
I found no example of such
I am just too short for fp16
I can run an AWQ quant of a 70b with 13k context on fp16 kv-cache. But those 2 billion extra parameters make it not fit at all
I am 900MB short, and going below AWQ is a significant hit in answer quality. I can get 14k context on fp8 with a 72B model, but at that point I have another choice: mantissa bits vs exponent bits
I currently run with 5 exponent bits and 2 mantissa bits. It visibly impacts quality. If i can get the scales, I can cut out another bit from the exponent and give it to the mantissa, while still being very close to a fp16 KV-cache
... it's completely insane, I've been working on this setup for years
Hmm
Years of /r/LocalLLaMA lol
I am still amazed that we can run something in our house that somewhat rivals cloud LLM's
Imma try the cache scaling
It's expensive on large models
Definitely make sure you have more than 2x system RAM vs your weight size lol
Don't make the mistake I made
Would you like my script?
Sure
Sure
(Proceeds to try ut on a A40)
installing flash-attn takes a long time
The MAX_JOBS I set is for the RAM I have. Might OOM your system
(it took over 100GB ram during compilation afaict)
A networked drive is super useful. You can use a CPU instance to download model weights into /workspace, and set up a virtual env to run the pip commands without eating precious GPU machine hours.
Probably because the venv is in a network volume
No it is just a stupidly compute heavy process. I didn't use the networked venv for it, hindsight is 20/20
It eats up a huge amount of RAM. Because of RunPod systems you see a huge amount of CPU cores available and this causes ninja to run lots of tasks, making you OOM, so MAX_JOBS is a must. I found that it ate 16 CPU cores consistently for 30ish minutes - hence I recommend the networked venv
you should install a prebuilt wheel
I couldn't find one that works
Maybe it's the B200
https://github.com/mjun0812/flash-attention-prebuild-wheels exists but is not for CUDA 12.8
GitHub
GitHub - mjun0812/flash-attention-prebuild-wheels: Provide with pre...
Provide with pre-build flash-attention package wheels using GitHub Actions - mjun0812/flash-attention-prebuild-wheels
u need a 4bit model with 8bit kv cache ig?
@JohnTheNerd (sort of) good news
I think it works with 1xA40
yep
how?
that won't even fit a single layer
bad news is
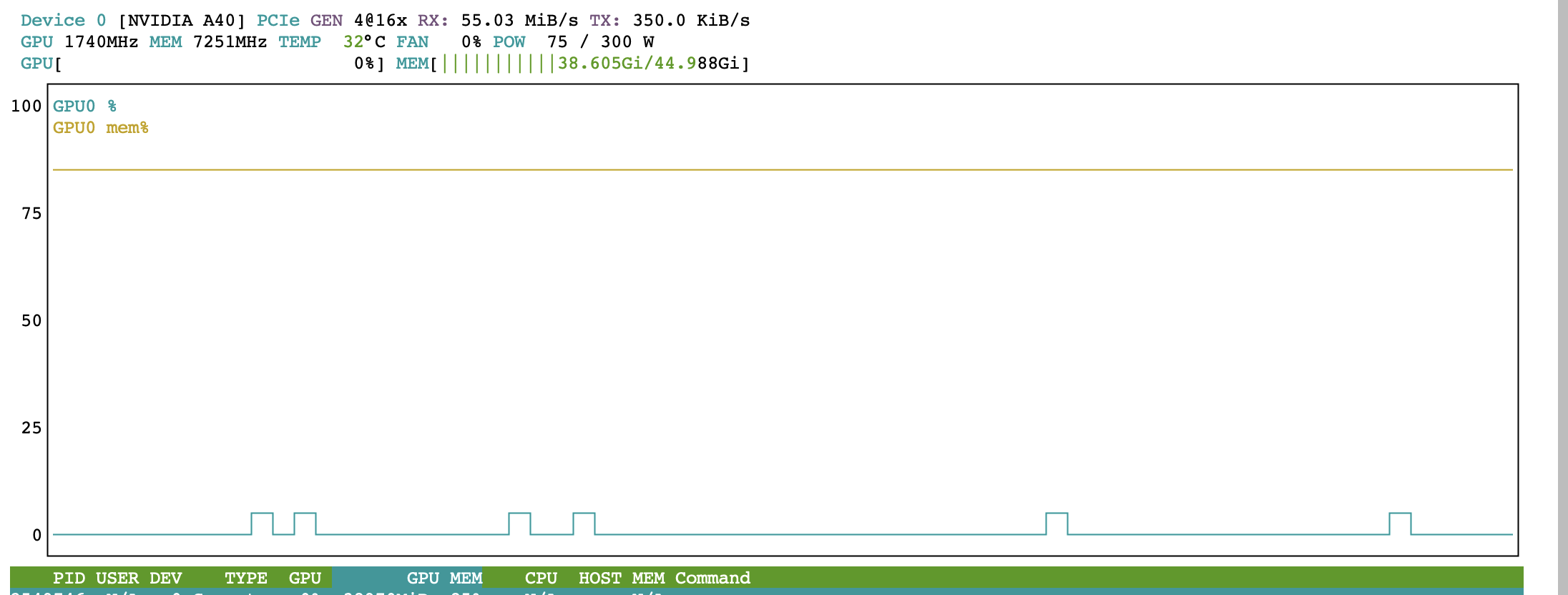
with CPU offload turned on
maybe it will work with your local machine
oh... yeah...
what's the speed like?
better(sort of) now
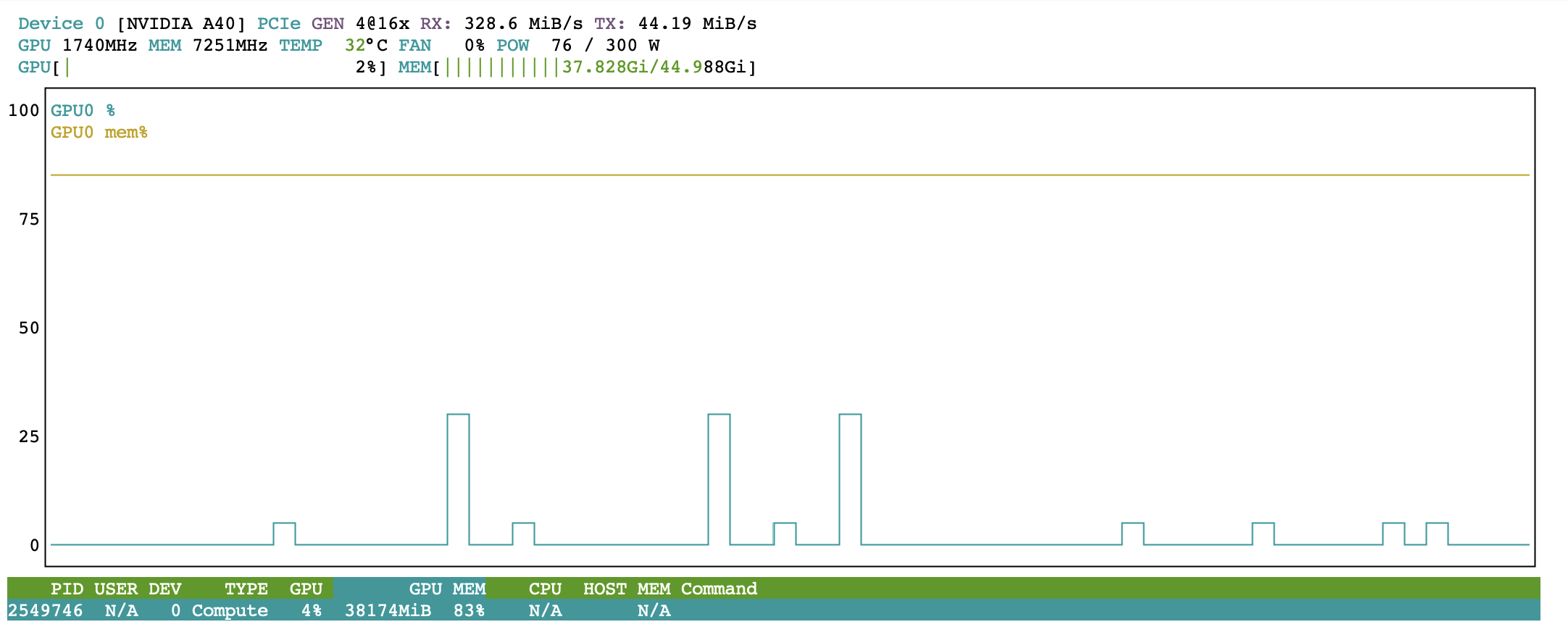
how many seconds per iteration?
idk cuz it didnt even complete 1 iteration
hahahahahaahaha
to be fair only only about 3 minuites has passed
assuming 5 minutes per iteration, that's... over a week for 2048 samples
maybe ill try with 4xA40s
lol
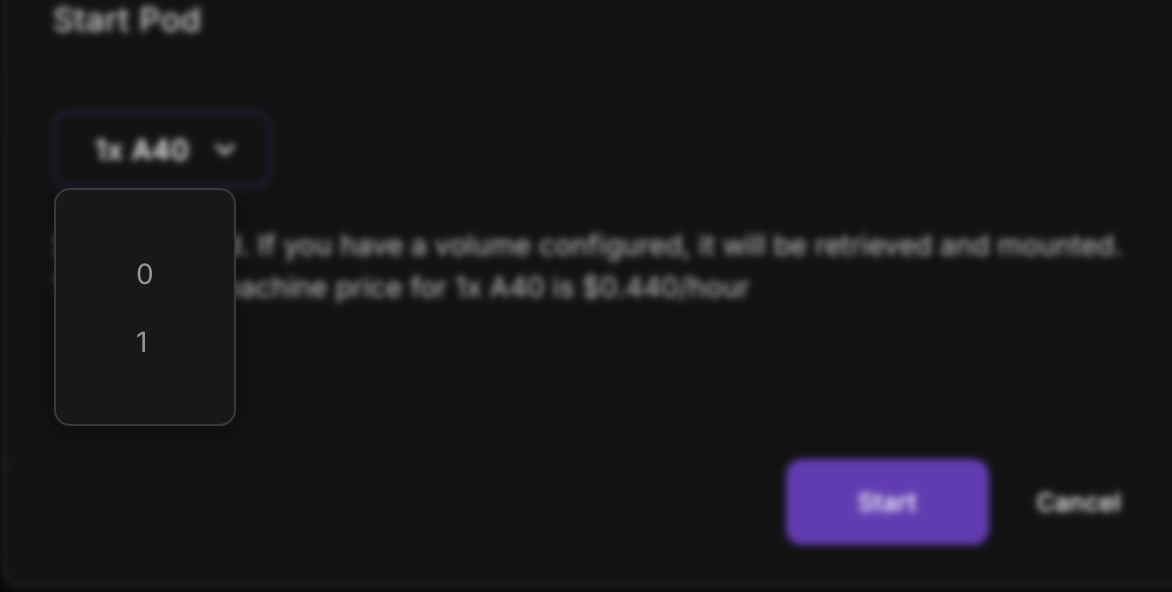
flash attention cannot run on meta device so it will be slower than that
Create a new pod
About 10sec per iteration with 4x 3090
5hrs
Total
that's actually really good
how much RAM do you get on that pod?
Try 4090
Im broke š¦
200gigs?
Im gonna pray for no OOM
I think you'll get an OOM
I had more and I got an OOM
What is the process that you're doing called?
I'm not sure it has an official name. I'm collecting KV-cache quantization scaling factors
the vllm link above has more information
Okay thanks!
seems like its this part
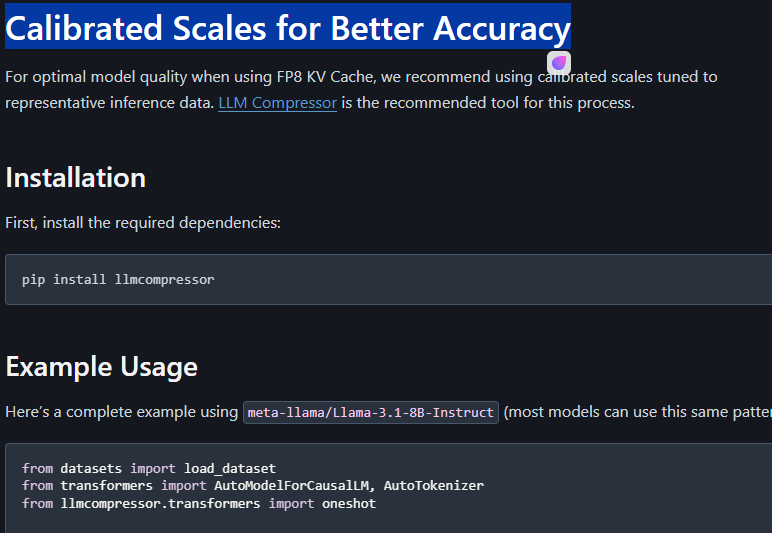
yes it is. but the code there didn't work for me. see my script above for what does work
at least until the OOM lol
Im trying with 32 samples
To see if it saves
that makes sense
the OOM doesn't kill your process. it freezes the entire pod
Okk
Currently praying
I'll get a pod of my own and keep trying once I get paid
until then, I'll go to sleep since I work in the AM lol
I cant save either because of a bug
it complains when a model is offloaded
trying this
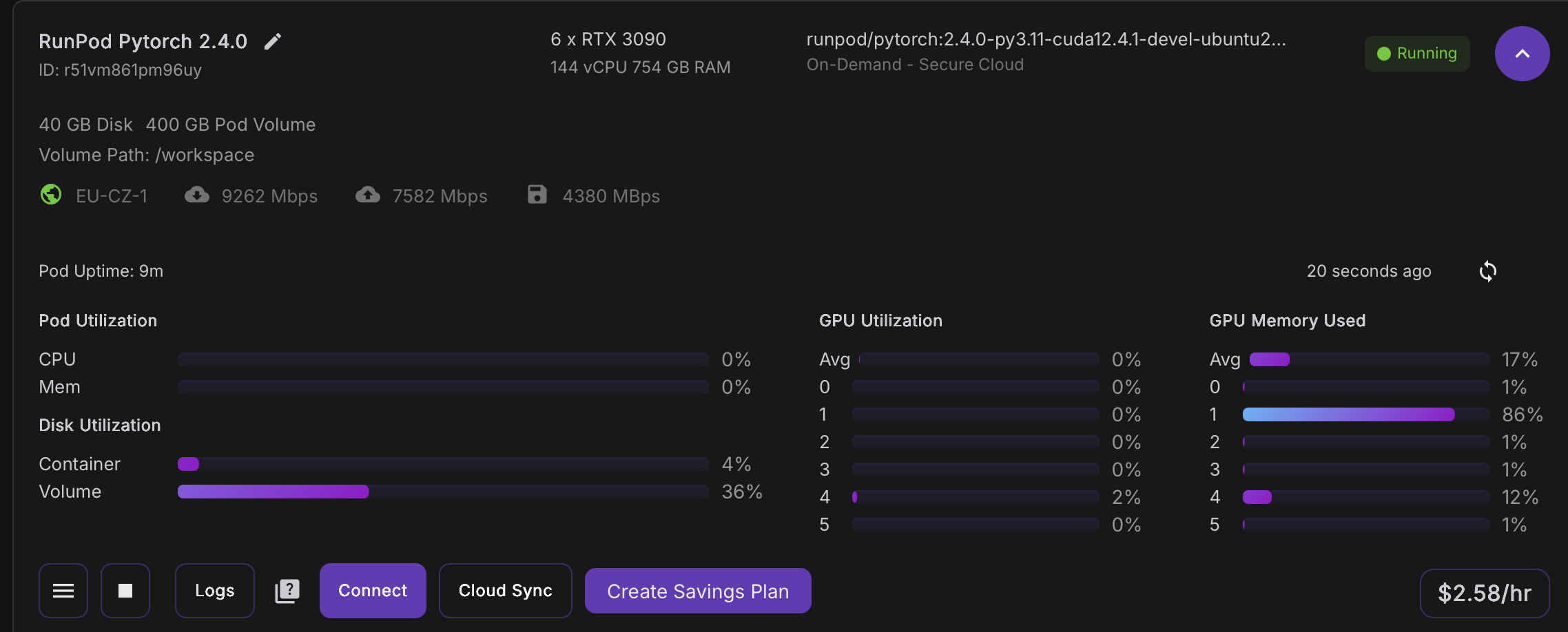
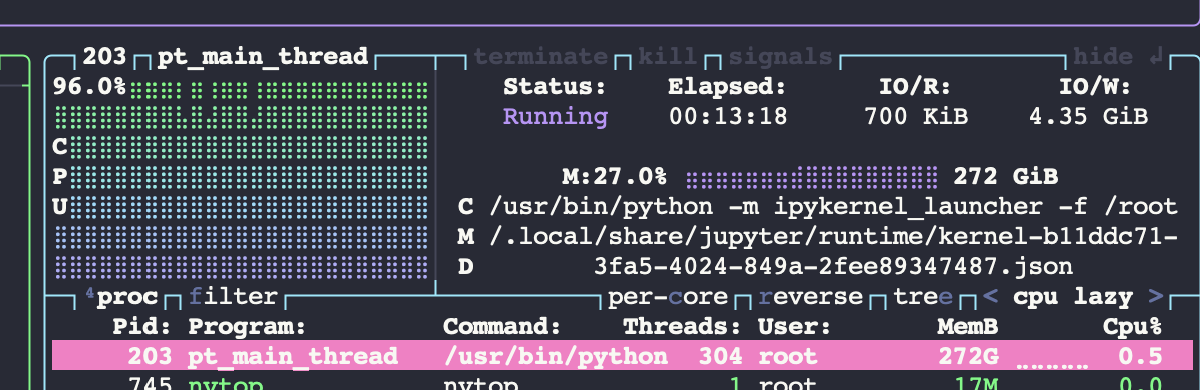
that is the maximum usage
so you probably needed like 10 more gigs of ram
š¦
š
is this all you changed for it to work with multi GPUs?
the code
and your model config(?) part is wrong
you need to quant it to 4bit int for it to fit in 2xRTX3090
I was just hoping to get the kv cache stuff. I use the AWQ quant because it's much much better than a straight 4bpw quant
maybe I don't even need to quantize the model lol
yeah you can do only kv cache quants
its on somewhere at the llmcompressor repo
in their test suite
GitHub
llm-compressor/tests/e2e/vLLM/recipes/kv_cache/default.yaml at main...
Transformers-compatible library for applying various compression algorithms to LLMs for optimized deployment with vLLM - vllm-project/llm-compressor
here
idk why they hid it so deep
that's sure hidden deep
interesting
thanks!
That's for tests.. Maybe it should be documented in vllm
if pod is using too much ram it will throw oom errors
it does not. it just completely freezes
The process doesnt get killed and just freezes the entire thing
Yup
With no errors
the entire pod simply freezes - no OOM errors. I do wish we could have swap in any way... Docker supports it, one would only need it implemented in the docker run command runpod executes. the fact that system RAM is limited by GPUs without any way of swapping is extremely limiting :/
You can deploy pod with higher ram
it's especially compounded by having a limit of 6 GPUs
Use filter option
even the B200 system doesn't have enough RAM for this workload. and the RAM is simply used once, at the end, not even continuously
the only way is to get 6 GPUs which is very wasteful when you just need system RAM
For B200 you can get 283 GB RAM
and even then, you cap out at some point. if you wanted to do this on slightly larger models, say Mistral Large, you're out of luck
yes. that was my pod, which froze
hence I wish there was some way to swap - RAM is expensive, swap is cheap. obviously I have to pay up for it, but paying for a second B200 hurts when all you want is RAM lol
If it's requires more than that it could be problematic. Not that simple as swap basically uses ssd storage causing faster were off
that's very fair - I appreciate the honesty
So in both cases there is technical loss. And usually people rent pods for GPUs with lot of VRAm š
I think I am the only person who needs both lol
it's because of such a stupid bug, too...
What kinda of bug? Tried submit issue on thier github?
model.save_pretrained tries to write the weights to RAM. twice.
you can imagine the joy it is to find that out with 150gb of weights sitting in VRAM
I'm guessing it's deep in the transformers library - which is what loads the weights initially. I suspect no chance in a GitHub issue being seen lol
Difusser? Or something else?
transformers
Cpu offloading breaks save too
also can't have flash attention with cpu offloading
my understanding from qwen 2 (not necessarily 2.5) is that it really, really likes flash attention
heard many reports of broken output without flash attention
So what are you doing?
Quantization of KV cache
To fp8
short version: I'm trying to get some magical "scales" to quantize my kv cache more optimally
With scale factors
GitHub
safetensor/mmap memory leak when per-layer weights are converted do...
System Info While working on GTPQModel which does gptq quantization of hf models and load each layer on to gpu, quantize, and then move layer back to cpu for vram reduction, we noticed a huge cpu m...
this requires me run inference on the whole model in fp16 thousands of times to calibrate a set of scalar
that's interesting but I suspect is not the issue I have. I don't have any issues moving weights to the GPU, and do not convert dtypes at all
the entire process runs just fine. right at the end when I call save, it eats the entire system RAM
Anyway late here so bed time for me
fair enough - maybe I'll get another pod today and try again with a lot more RAM this time
I'll post here how it goes lol
8x 3090
100percent works
can you get 8?
i thought cap was 6
Cuda oomed it thi
huh
I'll give it a shot
oh
cuda oom'ed it?
So 8 should work
Yeah for 7x3090
oh ok that's during weight loading
also I'll have flash attention which saves a bit
No it happened while quantizing and i had flash attn on
huh, ok then 8 should work. lots of RAM too
Use the wheels here it works well
perfect thank you!
No build time magic
š
I'll share the scales if I get it working
I wasted at least an hour of B200 time on just this lol
only 4090 can give me 8 at a time it seems. still workable - and a whopping 880GB RAM which should definitely be enough
Yeah you beed about 300gigs
*need
btw why do you use GPTQModifier in the quant instead only kv_cache_scheme
im using this now
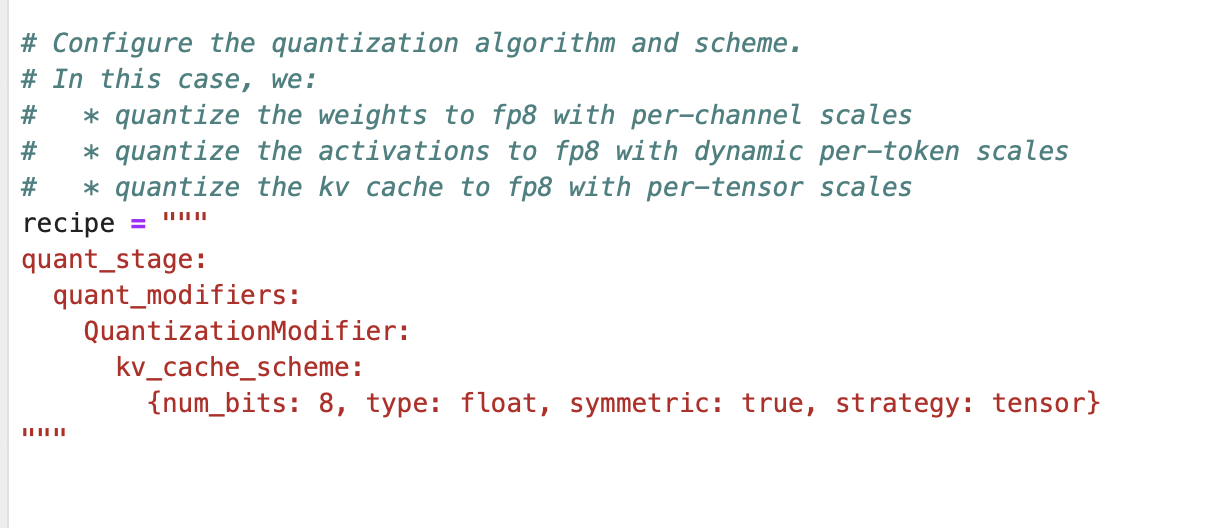
he uses AWQ which llmcompressor does not support
GPTQ was just for testing
ohh ic thanks
oh now to fp8?
what model are you doing
kv cache to fp8 weights to int4 (with AWQ)
Qwen2.5-72B-Instruct this one
ooh is the process still running?
Yes
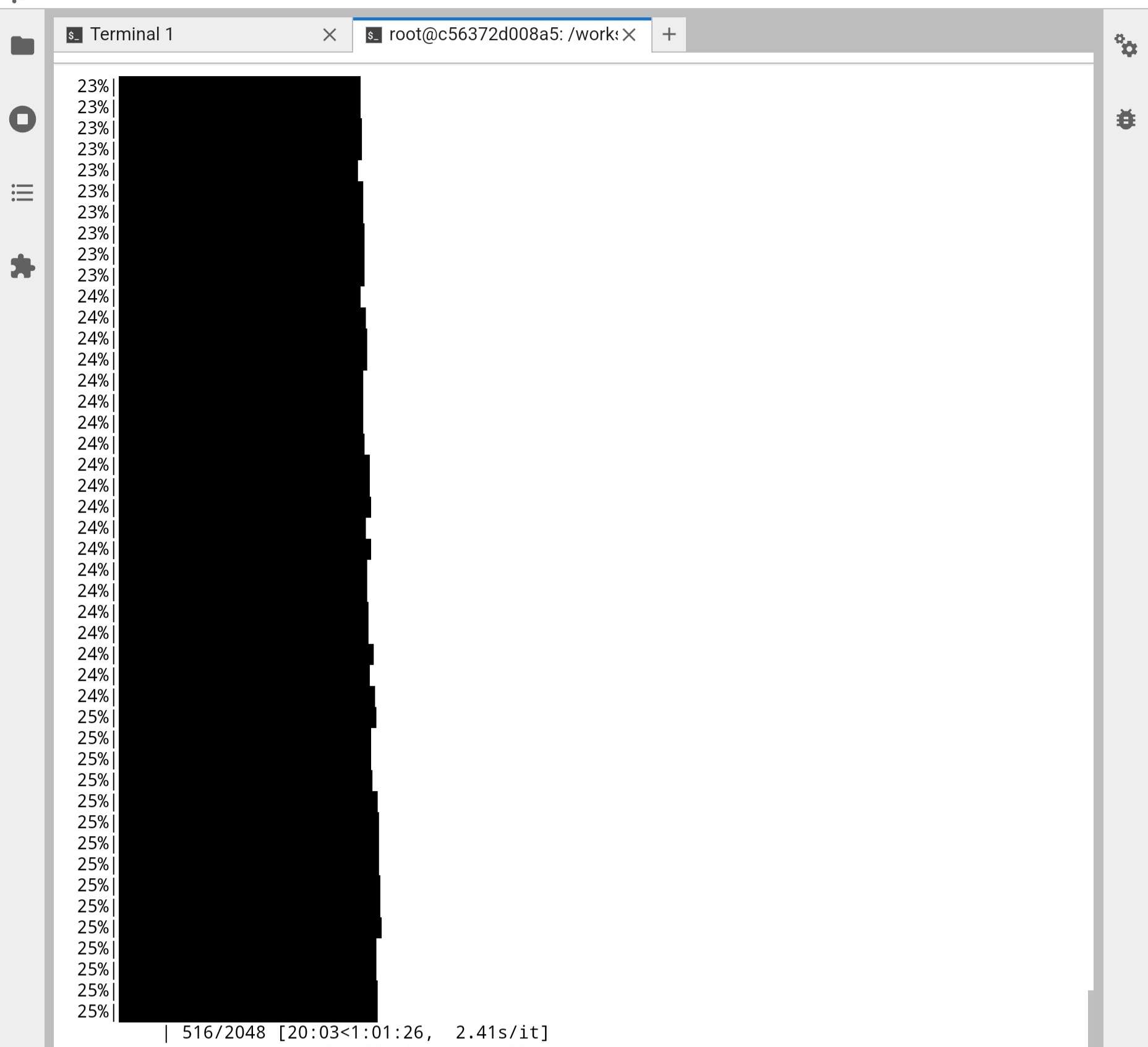
ah using what gpu?
8x Asomething
With 24gig vram
ohh a5000*?
I think thats right
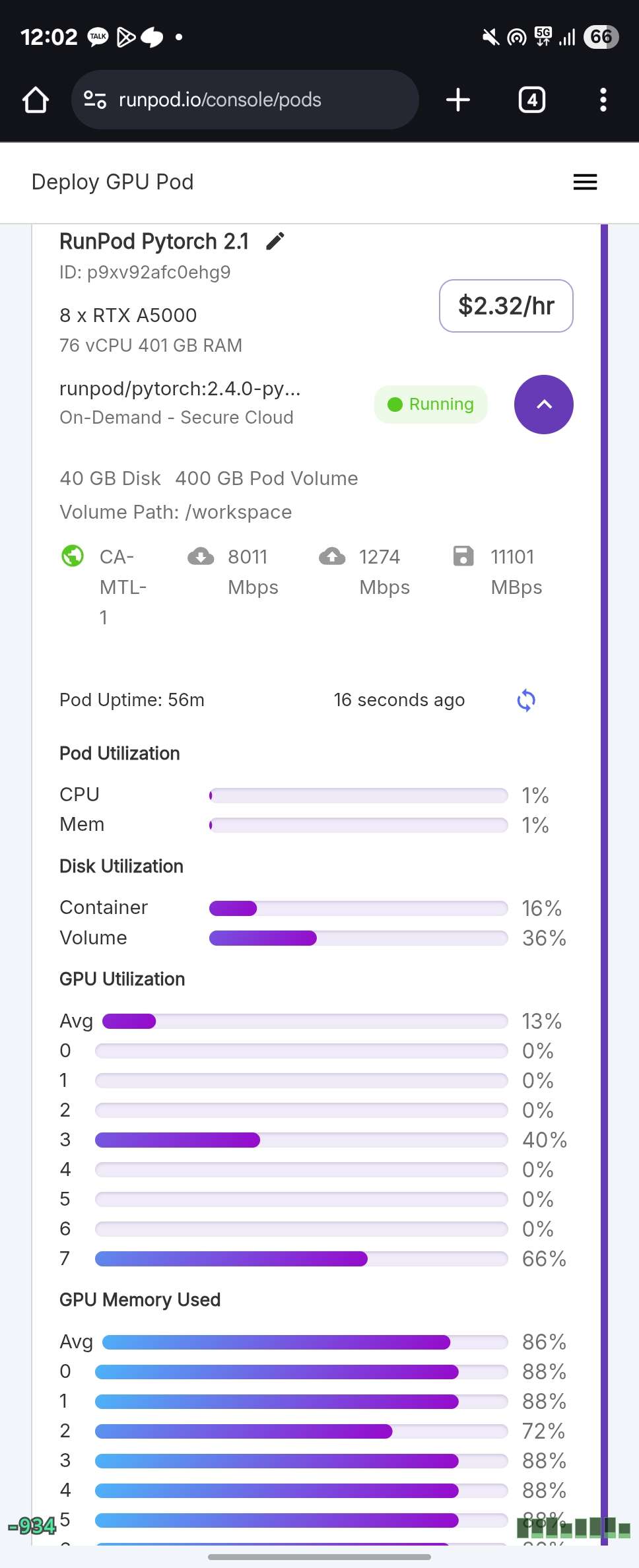
Seems like a good deal
actually its not my money š
Ohh
That's nice
its saving now
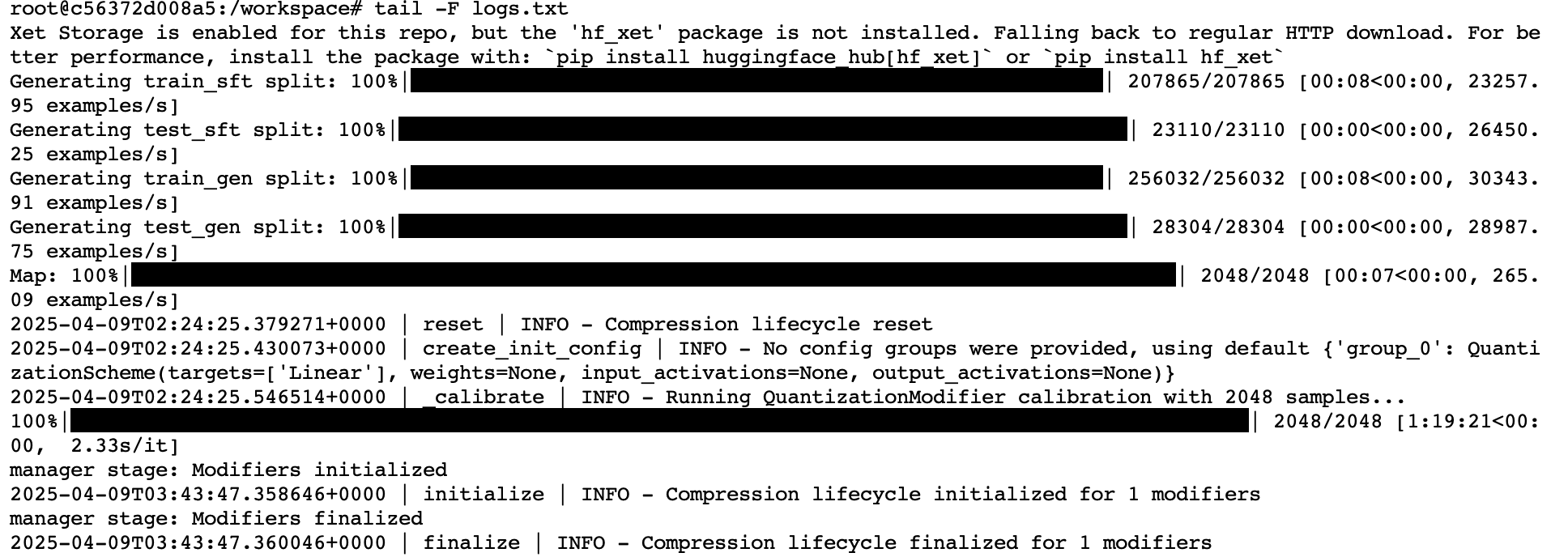
Wohooo
will you publish it
how long did it take in total
yes
maybe about 1.5hrs?
oh quite effecient
it says 1hr 20min for quantizing only
pod uptime is 2hr due to model downloading and installing dependencies
ic
and bc of my stupidity
yeah still faster than a few hours in b200 wow
i selected the wrong wersion
of pytorch
š
saving takes a lot of time tho
./models/Qwen2.5-72B-Instruct-W4A16-FP8-KV this should be
./models/Qwen2.5-72B-Instruct-FP8-KV
whats the difference whats the second one?
so who paid for this run haha
W4A16 means weights quantized to 4bit and Activation 16bit but i didnt quantize any
my (previous) school ig?
woah
got about 500$ for research funds
ig? why i guess
noicee
technically schools property but only i can use it
hahah okay i see
its writing to disk now
almost done
ooo awesome! i just filled my runpod account with 15$ without checking lol
I can do another model for you if you want
its finished now
āŗļøAnother time hahah
meanwhile I did something that may be useful. I'm running a benchmark suite on my qwen setup. I will re-run it with the scales too
Did you estimate after this quant it'll run on your home server or what
unfortunately because it's local it's slooooow lol - 12000 prompts to run on two 3090s
I'm already running fp8 kv cache - just with e5m2
that's what benchmarks are running on
Oh is it a bigger version of this?
no
quant_stage:
quant_modifiers:
QuantizationModifier:
kv_cache_scheme:
{num_bits: 8, type: float, symmetric: true, strategy: tensor}
is this right tho?
yes it is
what does symmetric mean
It's fp8 kv cache too?
yes
e5m2 is one sign bit, 5 exponent bits, 2 mantissa bits
And this?
e4m3 is one sign bit 4 exp 3 mantissa
you can choose to do e4m3 instead. but exponent in a float determines the range of numbers you can represent, which makes it awful
this helps e4m3
I have the response from openai's model if you want lol
I don't know what symmetric is so I'm curious too lol
So this one is e4m3?
Explain what's the effect of the exponential and mantissa amount lol
the idea is that you can try to have a list of numbers you multiply the kv-cache entirely by. this lets you get a little closer to fp16 even with the less range
let's consider a floating point number.
-35x10^6
the minus is the sign bit. plus or minus. we're left with 7 bits
the 35 would be the mantissa. and the 6 would be the exponent
(roughly, so)ā strategy: tensor
āāāāIndicates that the quantization is applied at the tensor level (as opposed to, say, per channel), meaning the same quantization parameters might be used for entire tensors.
āāāā dynamic: false
āāāāThis means that the quantization parameters (such as scaling factors) are fixed after calibration rather than being computed on the fly (ādynamic quantizationā). Fixed parameters can sometimes lead to more stable behavior.
āāāā symmetric: true
āāāāSignals that the quantization should be symmetric around zero. In symmetric quantization, the āzero pointā is fixed to zero, and the range is symmetric (e.g., āX to +X). This can simplify the arithmetic and sometimes improve performance.
if I only have two bits for the mantissa, I cannot represent 35 anymore. I must round it to a number I can represent
ic, still hard to understand maybe im lacking the bits thing, isok letme chatgpt it to dig deeper
thanks!
the 6 is the exponent. this effectively determines the range in which I can represent numbers - as I cannot say, have a 10^500 with a 4-bit exponent
since 500 doesn't fit in 4 bits
oooooo
thank you!
I'll try it out after the benchmarks run
wow in s3?
thats only the log and code files
uploading the model now
i thought it was the model š¤£š¤£
no way 140gigs is uploading that fast
yes way, lucky connections
can I have the kv scales? should be much smaller
wait you can do that?
so
the operation we are doing doesn't actually care about model weight outputs
to explain I must go back here
the output isnt the whole model? is just the scales?
yep
the scales are basically a lot of numbers
say I happened to have a lot of GPU power. I can run everything with its full precision for a little bit, paid by the hour
how tho
i saved the full model
it's just a json file
kv_cache_something i think
kv_cache_scales.json
https://docs.vllm.ai/en/v0.6.3/quantization/fp8_e4m3_kvcache.html
ahh i see
I would run thousands of prompts
I would check how much that fp8 kv cache actually differs from the fp16 kv cache
and come up with a set of numbers, when multiplied with parts of the fp8 cache, get as close to the fp16 versions as possible
those are my scales
in short batch processing-like right?
it didnt save that
maybe its fused
correct
interesting
yeah because the code is like
means its saves the whole modified model isnt it?
yep, that's right
yeah
I found this
GitHub
vllm/examples/fp8/extract_scales.py at v0.6.6 Ā· vllm-project/vllm
A high-throughput and memory-efficient inference and serving engine for LLMs - vllm-project/vllm
GitHub
[Bug]: The FP8 models and FP8 KV-Cache-Scales loaded together faile...
Your current environment Collecting environment information... PyTorch version: 2.3.1+cu121 Is debug build: False CUDA used to build PyTorch: 12.1 ROCM used to build PyTorch: N/A OS: Ubuntu 22.04.3...
I believe they extract it from the model
but yeah that isn't using the llmcompressor, yeah nvm don't really know how this extractor thing works
llm-compressor is by vllm too. I suspect it'll work fine
what happens if you don't set compressed=true I wonder
I think It doesnt use compressed tensor format
I see
in any case I will take a better look at the weights tomorrow - I should go to bed it's 2am here lol
I suspect it's uploading on your end anyway
Yeah
GitHub
llm-compressor/src/llmcompressor/args/README.md at c5dbf0cdb1364c40...
Transformers-compatible library for applying various compression algorithms to LLMs for optimized deployment with vLLM - vllm-project/llm-compressor
I think it is fused
Got an error related to k_scales when saving with cpu offload last time
ugh, any ideas how to extract it out?
riverfog7/Qwen2.5-72B-Instruct-FP8-KV
yep, it's fused
"model.layers.14.self_attn.k_scale": "model-00006-of-00031.safetensors",
I'll look in detail tomorrow
okay so
yes
have to extract that
š
this can be extracted
just a pain
how about just quantizing the model to int4 with fp8 kv cache and loading that instead
I could do that but I suspect it'll reduce quality significantly
I have a different idea...
I'm thinking of just taking those scales and injecting them into the safetensors file for the awq quant
throwing that all in
AWQ is nice because it relies on calibration to determine the most important 1.5% of weights. then it leaves those at fp16, quantizing the rest to int4
maybe a support thread in the runpod discord isn't the best place to discuss this tho lol
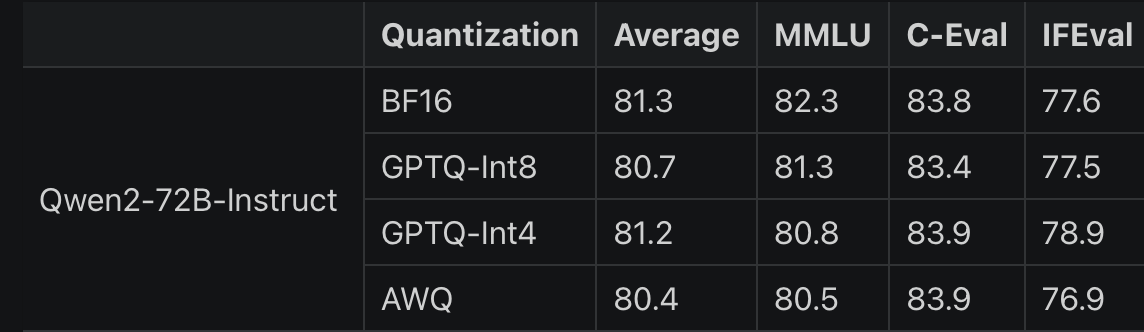
its actually better than AWQ
interesting
I should try it
its for qwen2 tho
and you can calibrate while quantizing the weights
that's true
like the kv cache
yeah
I'll do that, yes
good thing I have the 17$ on my account lol
that should be way more than enough
I don't see the scales in the model index, isnt it just a index referencing layers to the model file splits?
I wonder how the scales file look like
yeah actual data is in the .safetensors file
Ic
Is there scripts to run these benchmarks
its from the qwen docs
i think there are some llm benchmarking software so maybe use that.?
I ma look that up and try that someday lol
btw this thread has become VERY massive
Hahah no worries right
Great job
KV cache calibration is finished
GPTQ quanting left
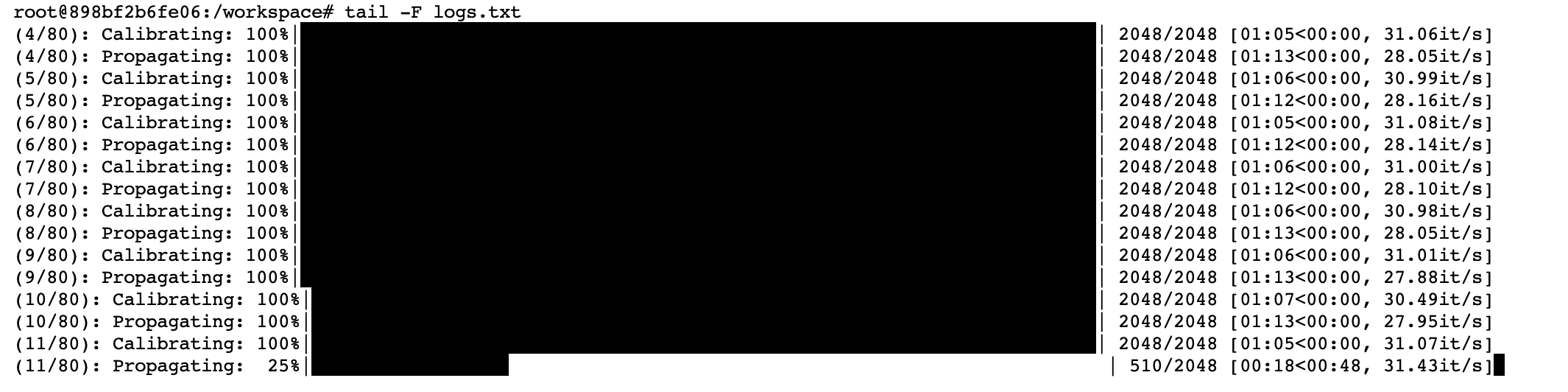
I think he will need more than 15$ for the quantizing
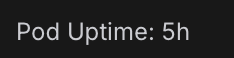
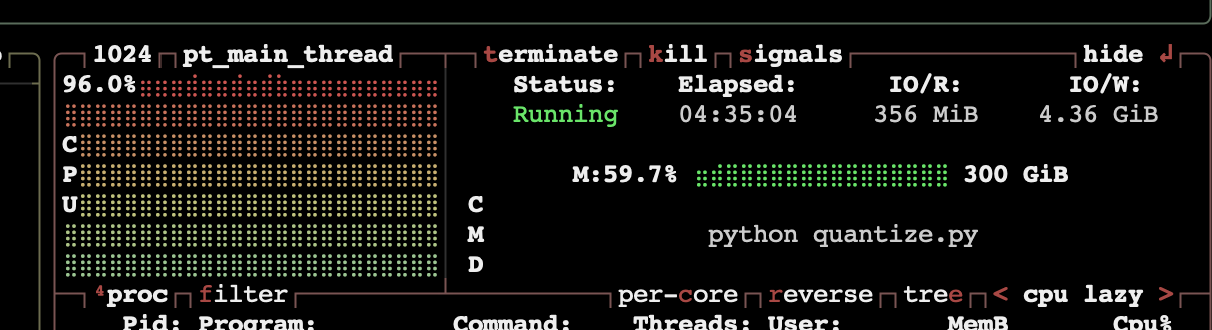
Btw why do you choose the gptq quant instead like fp8 or anything else
his gpu is 2x3090
fp8 doesnt fit in that
int4
and the benchmarks said that gptq 4bit performs better than awq so
i went with int4 weights fp16 activations with GPTQ
its int4-W4A16
oh the int4 is using gptq in vllm docs
idk about that tho
there's two types of quantization methods in llmcompressor
i see
hmm what is the other one?
QuantizationModifier and GPTQModifier
meta's ai is free in whatsapp wow
idk whats the difference but i used GPTQModifier
i think gptq is more complicated
okay its finished
finally
Yay
fuck
disk quota exceeded
Oof
Delete some other model
(needs to wait another 5 hours)
Hmm What for?
You mean repeat the process?
yeah
its on a py file
the process got killed
The importance of error handling š
that's sad
Im on 2xH200 now
much faster
iterations per second instead of seconds per iteration
Hahah
about 1hr left
i hate myself
It sort of finished
but why is the safetensors file size simillar to the original model if it is a 4bit quantized model
something's wrong
Howd you save it
it saves by itself
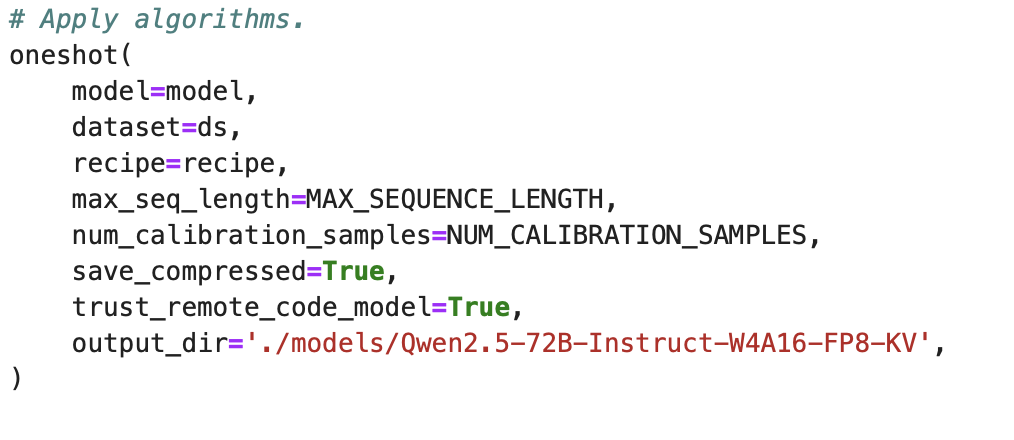
the recipie
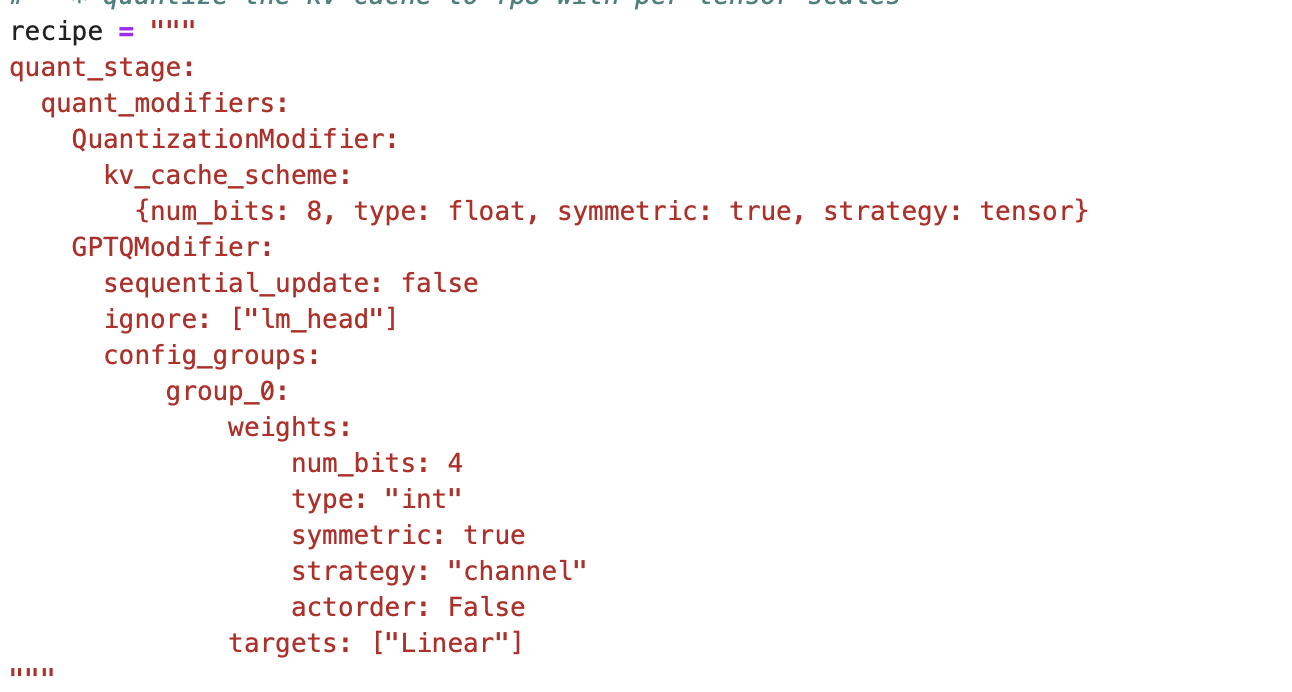
Ah try load it then
after uploading
ill try loading with 2xA40
should work
that doesn't sound right
that quantization config looks wrong
its this
I failed to figure it out
lol
Lol